Unlocking the Future: Emerging Technologies in AML Software
In the complex global of finance, staying one step ahead of illicit activities demands a blend of vigilance and technological innovation. As Anti-Money Laundering (AML) policies hold to conform, so too have to the gear and technologies designed to fight monetary crimes. In this journey, emerging technologies, appreciably artificial intelligence (AI) and device studying (ML), have emerged as the unsung heroes in the fight in opposition to money laundering. Let’s embark on a journey through the transformative impact of these technologies on AML software programs and explore a crucial addition – Data Cleaning Software.
AML regulations, the beating heart of financial security, are constantly in need of adaptation and evolution. Because money launderers are skilled at finding weaknesses in systems, regulations must be applied with flexibility. This blog explores how emerging technologies, in particular AI and ML, are woven together and how they support AML software in the face of constantly evolving financial crime.
The Foundation of AML Software
To draw close to the importance of rising technologies in AML, it is essential to recognize the traditional foundation of AML software programs. Historically, rule-based total structures laid the foundation, operating on predefined styles. However, the restrictions of such structures have become glaring in the face of the dynamic, ever-evolving nature of financial crimes.
Artificial Intelligence: Powering AML Revolution
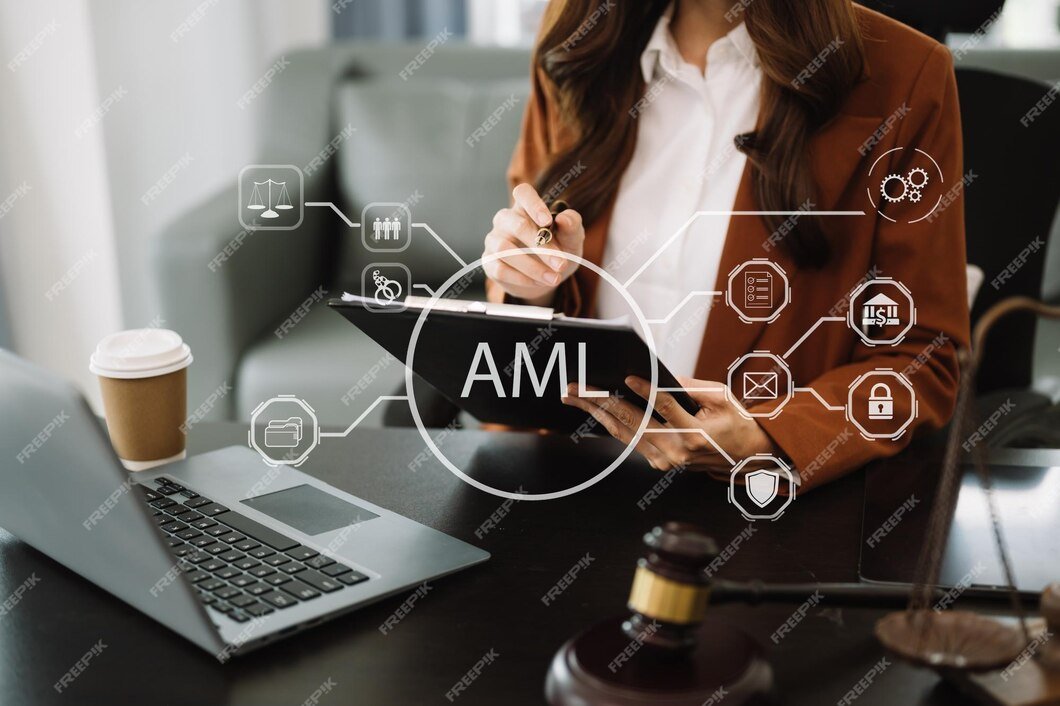
Understanding AI in AML
AI isn’t just a buzzword; it is the force behind the AML revolution. Its ability to investigate enormous datasets at unheard-of speed and accuracy surpasses human capabilities, forming the backbone of subsequent-gen AML software.
Machine Learning Algorithms
Machine gaining knowledge of algorithms, the brainchild of AI, brings adaptability to AML. Decision timber, random forests, and neural networks continuously learn from facts, adapting to evolving patterns and improving detection performance over time.
Natural Language Processing (NLP)
In the vast ocean of unstructured records, NLP acts as a compass for AML software. It allows machines to recognize and interpret human language, facilitating an extra nuanced analysis of facts from sources like news articles and prison documents.
Real-Time Transaction Monitoring
Dynamic Behavioral Analysis
In an international where transactions arise at the velocity of concept, AI and ML permit AML software to monitor transaction styles in real-time. Detecting deviations from regular conduct is paramount for the early identity of capacity money laundering activities.
Anomaly Detection
Machine getting-to-know algorithms excel at identifying anomalies in transaction facts. They scrutinize patterns, flagging suspicious activities that would slip through the cracks of traditional rule-based structures.
Enhanced Customer Due Diligence (CDD)
Risk-Based Approach
Gone are the times of 1-size-fits-all hazard evaluation. AI-pushed danger evaluation fashions now provide a nuanced and correct evaluation of patron hazards, bearing in mind a more targeted method to Due Diligence.
Continuous Monitoring
The evolution from periodic evaluations to continuous tracking is an instantaneous end result of machine studying algorithms. By imparting ongoing assessments of purchaser conduct, AML software will become proactive in figuring out potential risks.
Explainability and Transparency in AI
Importance of Explainability
Addressing worries related to the ‘black-field’ nature of AI algorithms, explainability becomes a cornerstone. Understanding the selection-making system is critical for building believe and assembly regulatory necessities.
Transparent Decision-Making
Advancements in AI are paving the way for transparent insights into decision-making methods. The collaboration among human analysts and AI structures will become extra effective while the decision-making intent is apparent.
Challenges and Considerations
Data Quality and Bias
The adventure in the direction of an AI-pushed AML utopia isn’t without challenges. Data first-rate problems and biases in schooling records pose good-sized hurdles. Strategies for addressing these demanding situations must be included in the improvement method to ensure fair and effective AML algorithms.
Regulatory Compliance
As generation advances, so do the rules governing its use. Adhering to regulatory suggestions is vital, ensuring that AI implementations in AML align with evolving felony frameworks.
Future Directions
Integration of Blockchain
The synergy between AI and blockchain holds immense ability. Blockchain’s transparency and traceability can supplement AI in improving the effectiveness of AML structures.
Collaboration of Technologies
The destiny lies within the seamless collaboration of rising technologies. The marriage of AI, machine gaining knowledge of, and cybersecurity equipment forms a comprehensive arsenal against financial crimes.
Data Cleaning Software: The Unsung Hero
In the realm of AML, the nice of facts is paramount. Enter Data Cleaning Software – the unsung hero making sure that the information fed into AI and ML algorithms is pristine. By figuring out and rectifying inaccuracies, duplications, and inconsistencies, Data Cleaning Software plays a pivotal function in improving the effectiveness of AML structures.
Conclusion
As we navigate the intricate landscape of AML, the mixing of AI and ML into AML software stands as a testimony to human ingenuity. These technologies, coupled with the regularly-not-noted Data Cleaning Software, empower corporations to reinforce their defenses in opposition to cash laundering sports. In this era of innovation, staying proactive and informed isn’t always just a method – it is a need.